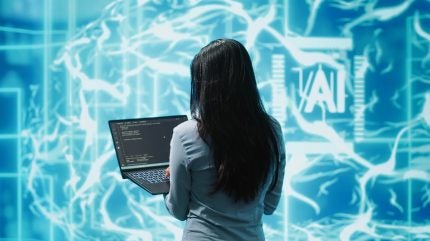
SandboxAQ has developed a dataset of battery performance and predictive AI processes in collaboration with the US Army’s Command, Control, Communications, Computers, Cyber, Intelligence, Surveillance and Reconnaissance (C5ISR) Center.
It has achieved progress in predicting battery shelf-life and maintenance for the US Army Combat Capabilities Development Command (DEVCOM).
This dataset will evaluate the status of lithium-ion batteries used across various Army applications.
Supporting the Army’s Power and Energy Modernisation initiatives, the dataset includes more than two million hours of lab and simulated testing of 18,650 cylinder cells.
AI simulations replicate real-world conditions such as battery ageing, temperature variations, and discharge rates.
This data, along with proprietary tools, will train SandboxAQ’s Large Quantitative Models (LQMs) to ensure lithium-ion batteries meet Army shelf-life standards, ranging from two to 20 years.
Traditional battery shelf-life estimation relies on proxy tests and extrapolation, which may not accurately predict performance after long storage periods.
SandboxAQ’s LQMs aim to reduce shelf-life testing time and enhance prediction accuracy.
In storage, these models can verify new batteries’ quality and shelf-life.
In the field, the company claims that future battery chargers could transmit performance data to the AI model, informing soldiers about battery lifespan and maintenance needs.
SandboxAQ AI & Quantum Application Technical lead Ang Xiao said: “Most commercial battery applications do not have the same rigorous performance or shelf-life requirements as those intended for military use, so most cell manufacturers do not take shelf-life into consideration when designing advanced battery chemistries or sourcing materials.
“The comprehensive battery dataset we’ve compiled with C5ISR Center will add this new predictive capability to our Large Quantitative Models, enabling all of our customers and partners to benefit from these previously unavailable insights.”
In October 2024, SandboxAQ reported that its LQMs cut the time to predict lithium-ion battery end-of-life by 95%, with 35 times greater accuracy and using 50 times less data than traditional methods.
This advancement reduces cell lifetime testing from months or years to days.
The results suggest that LQM-informed models could shorten new cell development timelines, saving manufacturers millions of dollars in research and development costs and accelerating innovation cycles in battery technology.